top of page
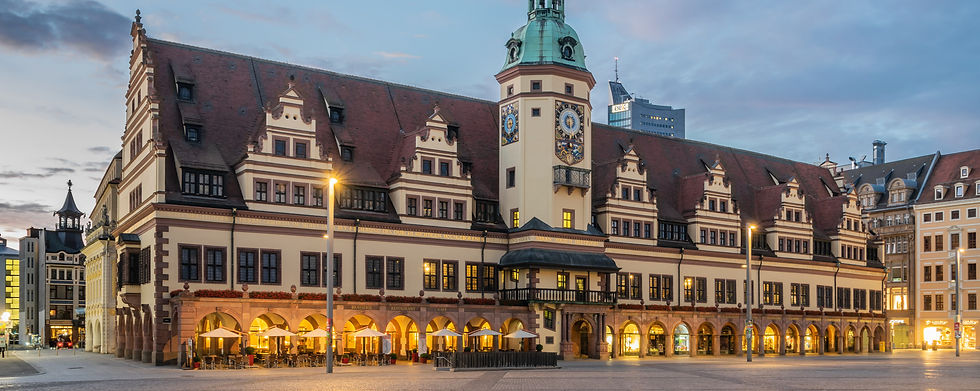_edited_edi.jpg)

ICDIS 2024
The 6th International Conference on Data Intelligence and Security (ICDIS 2024)
November 25-28, 2024; Leipzig, Saxony, Germany
Plenary Speakers
to be done!
bottom of page